Inspector's role in machine learning powered inspection
I just got certified as level 3 in ultrasonic testing, which got me thinking. What kind of certification should an NDT inspector need while conducting inspection with AI/ML assisted method. Should the inspector know how machine learning models operate, or how they are made? How in depth should the requirement go? Are certification even no longer needed with AI/ML models? And lastly of course, how do these AI/ML methods affect the life of the current and future level 3s.
For traditional level 1, 2 and 3 certification, the physical fundaments are taught fairly thoroughly, regardless the technique used. This is justified as understanding these physical phenomena are imperative to conduct a sound inspection. As we all know, you need skill, good equipment and knowledge in order to succeed in any inspection. Even if one of these is lacking, the performance decreases rapidly. For level 3 certification, broader view of the whole NDT field was required, including the manufacturing methods. While my theory side of things might be on solid ground, the actual practice, especially on the industry side needed some addition. I found this especially insightful as it gave perspective how the flaws are formed and what aspects should be considered more thoroughly regarding the manufacturing method.
Just like the broader view of level 3, many different techniques, such as phased array, Time-of-flight diffraction and digital x-ray, require additional training when following the ISO 9712. Even for level 3s in digital radiography. This is highly justifiable as the inspector's responsibility is to setup the device, conduct the inspection and evaluate the success of these operations. Every single step is paramount to understand as a single mistake in the setup might lead to missing of critical flaws. Therefore, the contents of these training days are fully focused on the physics behind the technique, as they should. When the rubber hits the road, inspector is alone with the physics, and its constraints, so might as well get used to these constraints.
So what about machine learning powered inspections? Should only level 3s be able to conduct these inspections. Surely not, they are few in number and already over worked as is. Extra training days for level 1s, level 2s and level 3s? Certainly, but not necessarily required in certification. I think AI/ML should be approached as a tool rather than a new technique. Currently, AI/ML is used as an evaluation tool, just like FFT in an inspection software. Naturally the inspector does not need to know the full spectrum what is happening in Fast fourier transformation, only the basics how to use it and what the result should look like, usually presented in the manual.
Just like any inspection software, the AI/ML gets the data when the physics have run its course. AI/ML model cannot rescue the data if there is no contact medium, not enough voltage or the film is misplaced. Expertise is needed, no matter how fancy a toolset you have. Moreover, there has happened a lot of development, testing and validation before any real inspection data is sent for the AI/ML model to be evaluated. While this is important and it is also equally important for the model developers to understand the inspection, this all happens in the background and the inspector has no real interface to affect these steps.
Therefore, AI/ML models and solutions should be designed in such a way that the model would help the inspector to evaluate the data and possibly rise a flag if the data is insufficient or lacking to perform the analysis. How the model reaches to this decision is inconsequential for the inspector. This would lead to the requirement that the inspector gathering the data should be at least level 1 certified. This would ensure that the one gathering the data understands the basics fundaments of inspection. The AI/ML model is a tool to make the work easier and efficient. Naturally if a flaw is found, level 2 or 3 will be used to make the final call.
Rather than having an effect on certification, I view the AI/ML models have the possibility alter the procedures for the better. Traditionally these procedures are large, describing how to setup the equipment how to analyse the data etc. Usually these procedures end up being so complex, that a separate NDT instruction is required for the actual practice of the inspection. Or in worst case scenario, the procedure might be impossible to follow. Setup phase can be highly automated, including calibration. Moreover, the indication data is pre-gated and presented the best possible way to the inspector. This reduces the burden on the procedure how to setup and evaluate the data during the inspection. Ideally, the procedure would remind more of the NDT instruction to level 1, making it more clear and straightforward.
Therefore, I would view the AI/ML models as a highly optimised inspection software, tuned for single inspection. Like an app on your phone. Naturally the operator should know the basics how to use the software, as there is no replacing the actual knowledge and expertise of a certified inspector. I for one intend to use this opportunity to write more direct and simpler procedures for the AI powered inspections.
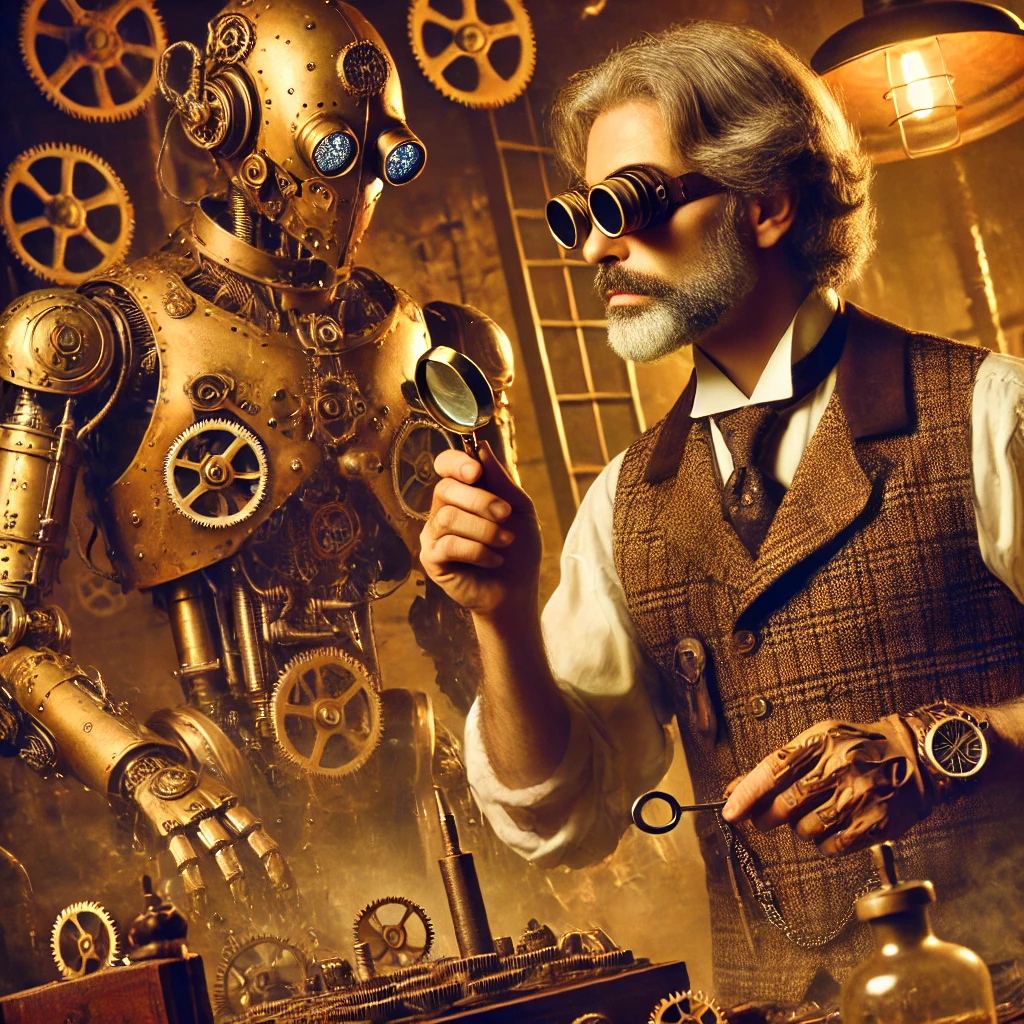
Another great field trial with EPRI on AI for NDE completed!
This time, the target was reactor pressure vessel visual inspections on a boiling water reactor. The visual inspections are a very significant part of the whole NDE scope, especially for the BWRs. Long hours in the critical path. Reliability and speed are paramount.
AI can help improve consistency, reliability and speed of evaluation. As we learned here, it can also help improve the coordination between the Level 2s inspecting and the Level 3s reviewing. When AI highlights region of interest, that cues the Level 2's to comment on these and helps the reviewers confirm that the features were identified and evaluated. For the utility, the AI helps focus review to the most important parts.
The performance was great, even with this being the first field trial on HD video. Real-time evaluation with intuitive highlighting of interesting features. The model successfully indicated all previous findings. Equally important: the model did not call too much to distract the inspector. The Trueflawbox once again proved it's legendary ease of use. Just plug it in between the video source and monitor and you are good to go. Connect it to your computer and drag-and-drop to evaluate previously acquired video files.
Kudos to Chris and Thiago from EPRI for making this happen, for Kathryn, Moises et al. from Framatome for superb vendor support and Oskar for all the hard work in AI training. Thank you for the site NDE team for welcoming us and supporting the field trial. It's a privilege and a joy to be working with you all!
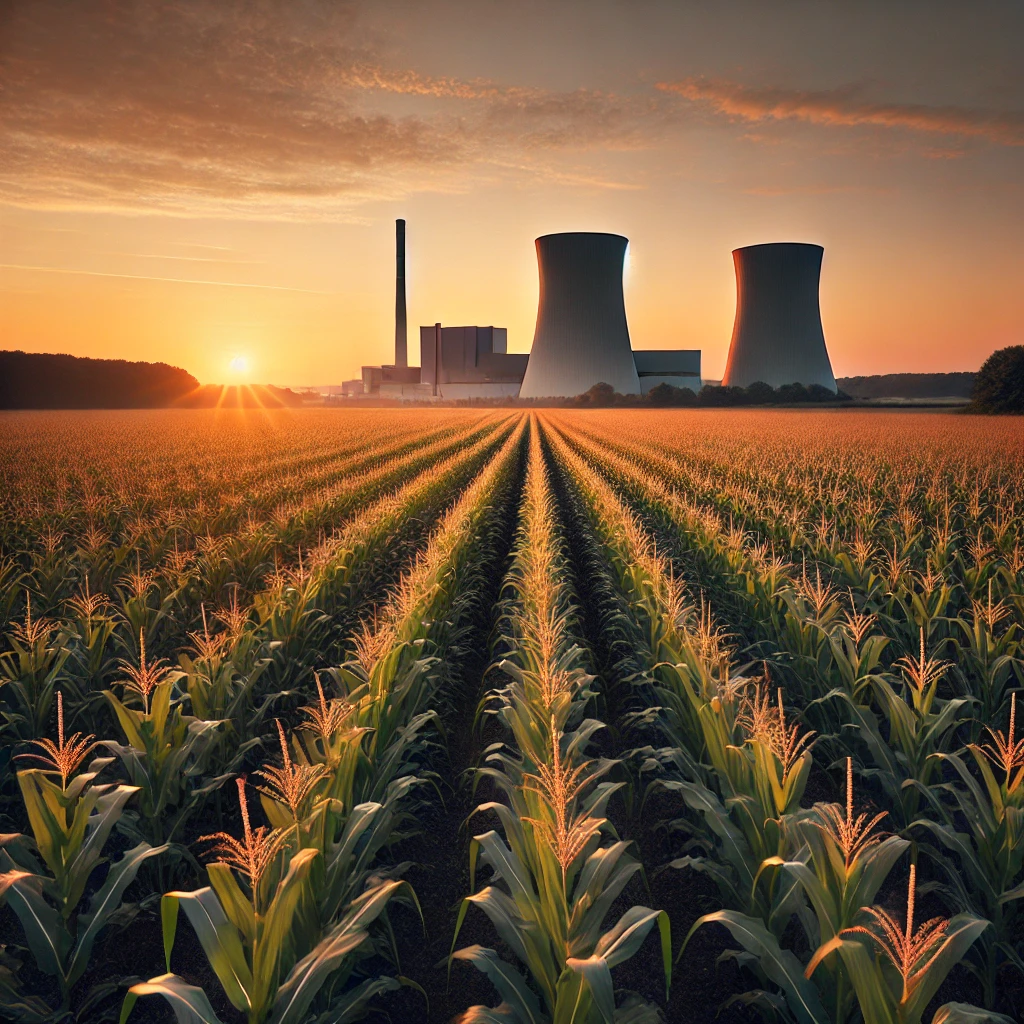
The EU AI Act was published last month. What does this mean to AI for NDE?
TL:DR: mostly, it will enforce best practices. Don't be put off by the term "high-risk". By and large, it just means that for applications with safety implications, you are mandated to have proper quality assurance and test your models. As you should anyway.
First, the EU AI Act is a remarkable piece of legistlation. It is easily readable (for the most part), and provides clear and measured regulation to address well described potential risks posed by some AI use cases. NDE is a specialized area and it is sometimes difficult to reconcile generic requirements with the specific challenges of NDE. I'm sure it's not the only such area. The AI Act does a really good job in taking into account such special areas and provides high level requirements while allowing specialization that makes sense for a particular implementation. It further highlights measured response and allows simplified implementation for small companies to avoid excessive regulatory burden. Special provisions are made so as to not hinder research and innovation. It's good news.
The AI Act broadly divides all AI use in three categories:
- Prohibited
These mostly include using AI as a tool to deceive or to undermine human rights or democratic processes. None of this is applicable to NDE. Do no evil with AI. This is the first part of the act to take force, 2025-02-02. - High-Risk (Regulated)
These are applications termed "High risk" in the Act. I think this is somewhat of a misnomer, since the Act provides measures to exclude and/or mitigate the potential risks. To me, a better word would be "regulated" use. At any rate, these are application areas, where the potential risk posed by the use of AI warrants additional regulation. Products in these categories will need to register as such.Much of these deal with the use of AI for biometric markers, surveilance, law enforcement, border control and privacy. That is, use cases bordering the prohibited area but with potential positive uses as well. These are not applicable for NDE.
The interesting section for NDE is the applications in regulated applications such as pressure equipment, aviation, rail transport, energy infrastructure, etc. are also considered, by default, high-risk applications. To oversimplify a little, if the use of NDE is regulated, the use of AI for that NDE will also be regulated (i.e. in the high-risk category), unless excempted (more on this later).
- Other uses (Unregulated)
As you might expect, the rest falls into unregulated space and the Act does not enforce you to do much here. However, the Act, and the accompanying draft emphasize, that it is strongly encouraged that actors would voluntarily take upon themselves to implement the maesures mandated for the regulated use cases. This may sound like wishful thinking from the legistlators, but I would concur. Most of the things required are just best practices that you should do anyway. Really, the only additional burden is the higher level of formalism and transparency that for high-risk use cases are mandatory. At any rate, if you're not following these practices, formally or otherwise, you're setting yourself up for failure.
So, where does NDE stand?
Is NDE a high-risk (regulated) application area? Yes, sometimes it is.
If the NDE is completed in absense of regulatory requirements, it broadly falls to unregulated use. For example, a lot of manufacturing inspections fall into this category, with primary objective being normal quality control and early detection of flaws to improve efficiency.
If the NDE is part of a safety function related to areas listed in the Act (≈ NDE is regulated), it will, by default fall into regulated use. However, it is still possible to use AI in a regulated application in a way that negates or effectively limits the potential risks associated with the AI and these are excempted. In particular, it's not "high-risk" if AI is used (list redacted and paraphrased):
- to perform a narrow procedural task;
- to improve the result of a previously completed human activity or
- to perform a preparatory task to an assessment for the use cases relevant for NDE.
Now, this maps quite well with the levels of autonomy in the ENIQ RP13, the upcoming IAEA tecdoc and other documents:
- if the AI is used to check human evaluation after the fact, its clearly unregulated use
- if the AI is used in full autonomous mode, it's clearly regulated use (this is not currently done or recommended anyway).
Where it get's a bit more complicated is, when the AI is used to screen data and defer areas of interest to human judgement. This is the current recommended approach and thus the application has special importance. I'd say this requires case specific judgement. In most such cases, the AI is used as part of a well defined procedure with guardrails in place to limit potential risk and used to reduce potential human errors by reducing the burden of evaluating voluminous benign data. Judging as a whole and for the intent of the Act, I'd say this constitutes a "narrow procedural task" and "preparatory task to an assessment" and so would fall to unregulated use. However, I can also envision a use case, where the NDE would be mandated, but the requirements are left fairly open and as such the AI could be used in a way that materially affects the results and would fall under regulated use.
In practice, I don't expect this to matter much. Regardless of whether the use case is regulated under "high-risk" and whether it's voluntary or mandated, I'd recommend implementing the measures required for the regulated use cases, as applicable.
What is required from the "high-risk" use cases?
Again, much of these are not applicable for NDE, such as provisions to deal with controlling personal information etc. The parts that are applicable for NDE mainly deal with the quality assurance, monitoring and evaluation of fitness-for-purpose of the AI system.
The AI Act requires the "high-risk" AI applications to maintain a quality system and version control the AI products put to market. It requires you to evaluate the used data for omissions, bias and other potential shortcomings and assess the potential impact of these shortcomings. It requires you to test the results of the trained models and complete solutions, and maintain test reports. It requires you to justify the metrics used in the evaluation to show they properly relate to fitness for purpose. It requires maintaining documentation to provide traceability of products, versions and training data. If pre-trained models are used, evaluation of these, possibly in mandated collaboration of the provider of such models. It requires a risk management system that includes evaluation of potential risks, mitigation strategies and following actual use, as applicable.
The AI Act also has specific provisions about the human-machine interaction. The AI systems shall be designed in a way that make it easy for the human operators to effectively use it. The functioning of the AI system is to be transparent to promote the operators using it as intended. The AI system shall be designed to provide for effective human oversight, i.e. the results shall be available and easy to verify for the human operators/deployers.
All these are things that you should do anyway if you're using AI for NDE. The only additional burder, is the required formalism: regulated "high-risk" applications need to register, they need CE marking and the associated declaration of conformance, the test reports need to be signed by responsible individual, etc. But even that does not seem particularly burdensome or excessive.
Conclusions
The EU AI Act will mandate quality assurance, traceability, transparency and registration of AI use cases that are regulated as "high-risk". Some of NDE use cases will fall into this category. Regardless of whether a specific use case is regulated, quality assurance, testing, traceability and transparency to inspectors should implemented.
Thus, overall the EU AI Act is good news for NDE AI. If anything, it encourages and/or mandates a level of quality that allows trustworthy use of AI tools and hinders bad quality products from entering the market.
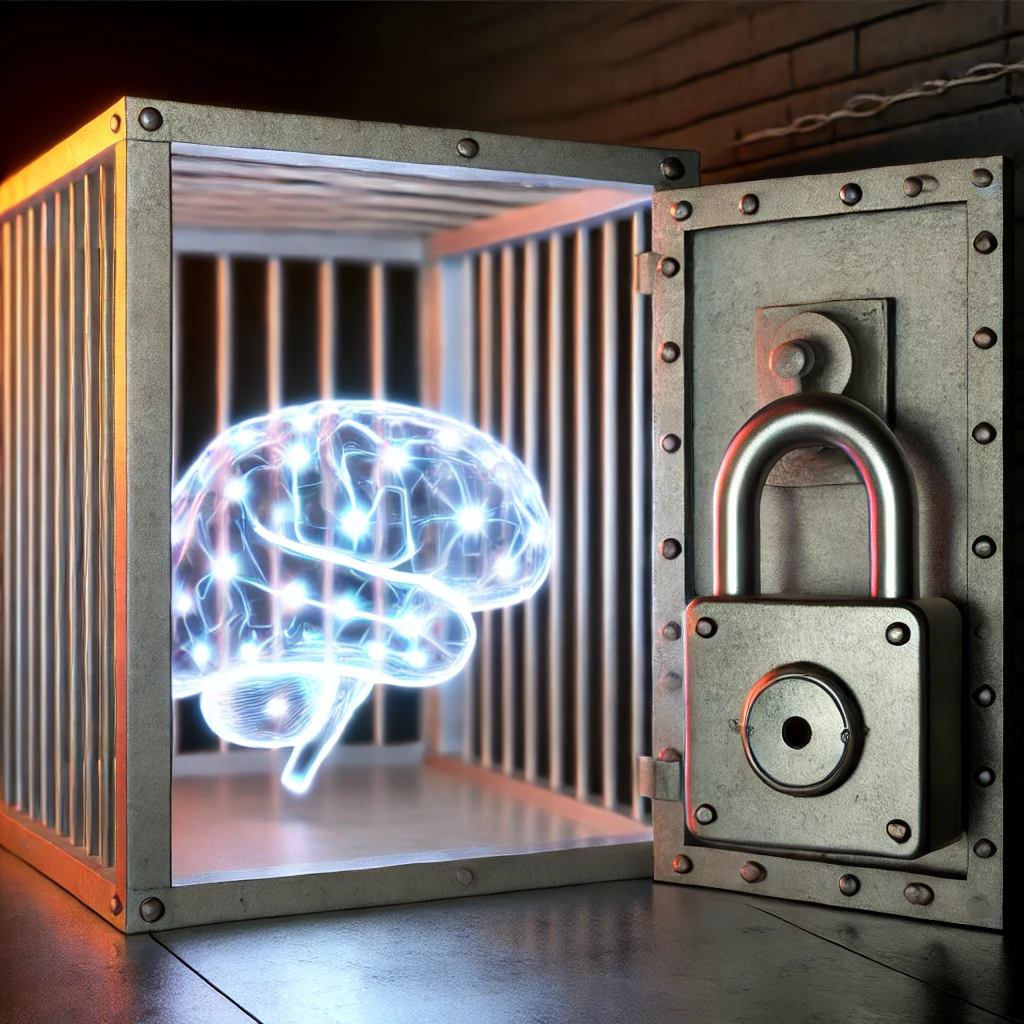
So you're using AI to evaluate NDE data. What does your model do, if something unexpected happens?
This is an important question and comes up every now and then. It came up at the EPRI TechWeek in June, and my immediate reaction was "this is not my favourite question". Not because the question stupid or unimportant – it's a good question. It's just that it's loaded with questionable assumptions and so the superficially simple question requires a longer answer to address properly. So, here's the proper answer. TL;DR: there's a solution and the concern is been addressed.
To begin, the issue is not as significant as it would appear and part of it is a case of saddling AI with requirements that the present systems do not fulfil. The first unstated assumption is that human inspectors are good at reacting to unexpected features of the data. This just is not so. There's a number of cases with unexpected flaws going unnoticed for extended periods of time. After first cracks are detected, suddenly similar indications are found in other plants. People in POD exercises who focus too much on the small flaws start to miss big flaws. The inspector's work requires high focus. The flipside is that we become blind to things outside of our focus. If you find this hard to believe, look at the famous monkey business illusion.
The second unstated assumption seems to be, that the AI is trained on random examples that just happen to result in good model performance, and so if a new failure mechanism appears, all bets are off, whereas for human inspectors this is somehow different. This is also wrong. The inspection procedures are highly tuned to detect known features of the flaws we aim to detect, e.g. the crack tip diffraction echoes for TOFD ultrasonics. The AI models are also trained to be sensitive to these specific echo patterns. I know the Trueflaw models are and I would cincerely hope this to be true for others as well. Now, asume the material finds a new way of degradation. This is a very rare event, but has happened. If this previously unknown mechanism features cracks, then the inspection method tuned to find cracks is expected to provide detectable signal similar to previously known cracks and the AI model is expected to generalize. Spoiler alert: most critical in-service degratation mechanisms feature cracks, which is why most inspection methods are tuned to find cracks. The signal might be a bit different, but it will necessarily retain the characteristic features of cracks. If the new degradation mechanism does not feature cracks, well then inspection method is not tuned for it either and chances are that there will be no signal to detect, AI or otherwise.
Thus, this question is of interest in a much smaller area than one would intuitively expect. It requires an unforeseen failure mechanism, unlike most degradation to date that the inspection method is, by chance, able to detect with a signal unlike typical flaw signals. So it takes a very rare event, on top of a second very rare event, on top of a third rare event to bring this question to bear.
Thirdly, the way it's often formulated, the question excluces a satisfactory answer. It's a trick question. If something unexpected happens, then, by definition something unexpected results. Otherwise you would not consider the thing that happened unexpected. As such, it cannot be answered, whether in the context of AI or otherwise.
All this notwithstanding, the question has merit, and the underlying concern is valid. It's not critical at present and should not prevent us from using AI today. However, as the role of the AI increases and the human inspectors review smaller and smaller portion of the data the rare events become more important. Also, it might not be a new degradation method, it might be an unlucky combination of dead elements or extenal noise that will not look like a flaw, but might hide flaw signals, if present. In all honesty, it took us a bit longer than I'd like to properly address it.
The key insight that allows a proper answer is a slight re-framing. The issue of data quality has been raised before, and we already have things in place to provide traditional data quality checks (IQI's in DRT, coupling control in UT, etc.) Re-framing this question as a data-quality issue, offers a proper solution. The requirement resulting from the underlying concern is not to predict the unexpected, which is impossible by definition. The requirement is to detect the unexpectedness, as with other data quality checks.
For traditional data quality checks, this takes the form of defining a metric and an allowed range, e.g. noise within bounds to confirm coupling. For AI, we need to have a metric to describe if the example is "expected" within the context of the training data. For this problem, we have a known solution: embeddings. With small change in the models, we can extract an additional output that provides a "fingerprint" of the present data. This can be compared to the training data and provides a metric of similarity relevant for flaw detection. This allows us to highlight out-of distribution samples and alert the use when the model falls outside validated range.
Long story short: there's a good solution and it is being addressed.
P.S. The data fingerprints have potential for other uses as well. They can be used to find potential duplicate data or similar conditions across plants to better support fleet wide condition monitoring. This is perhaps a good topic for a follow-up posting.
P.P.S. This solution is specific to NDE and will not solve issues we don't have, like adversarial input. However, the approach does hint a solution for those issues as well.
Can I use AI, when I don't understand the math behind it?
In discussing AI with various inspection vendors, one concern that is sometimes raised is that they are afraid they don't understand enough about AI to use it reliably. This is an understandable and justified concern, but the solution is not always intuitive. Should they take a course in AI? Should they refrain from using models, if they cannot do the math themselves?
My take: this is not the understanding you need. The AI courses are not going to help here. They might be interesting in their own right, but even if you become an AI professor, this will not provide the understanding you need to safely use them in an NDE setting. For this, you'll need a different kind of understanding.
The analogy I sometimes use is that of car racing. The mechanic will need a thorough understanding on the car to tune the car to be a winner. But this will not make them a good driver. The driver will need a thorough understanding of the car behavior to be a winner, but this will not make them a good mechanic. Neither one will win races without the other.
So it is with AI and NDE. The AI vendor will need to understand the mechanics behind AI to pick the right models and to train a robust solution. They'll also need to know quite a bit about NDE and the inspections to avoid pitfalls. This will still not make them inspectors.
Likewise, the inspectors will need to combine their experience and NDE knowledge to exercise the model and to build working understanding on how it performs under various conditions. It's certainly important to establish good communication with the AI vendor to support building this undertanding. However, trying to be both the mechanic and the driver is definitely not necessary and is in most cases infeasible. AI for NDE has proven to be quite challanging. Many have tried, but there's just a handfull of teams worldwide that have shown success. Many companies have started AI projects with high hopes and are still waiting for deployable results.
The good news is that the inspectors do this quite naturally and intuitively. Always, when we deploy a new solution, we find the inspectors eager to try it out and to exercise it on various data sets they have tucked away for special interest. Sometimes even with data sets that the models were not designed for and would not be expected to work with (open communication with the vendor is important here).
The sad part is, that they sometimes feel that this is somehow not enough. They may feel that they would be need to learn something new and completely outside their experience.
There's no need for this. The inspectors already have the skills they need to evaluate new tools. All they need is some hands-on experience to build this working understanding and trust. Work with your AI vendor to create safe environment for such experimenting. Evaluate model performance jointly on your specific setting. The vendor will also want to work with you to make sure they are delivering a solution that performs well for you. Build a strong connection with your mechanic, that's how you'll win.
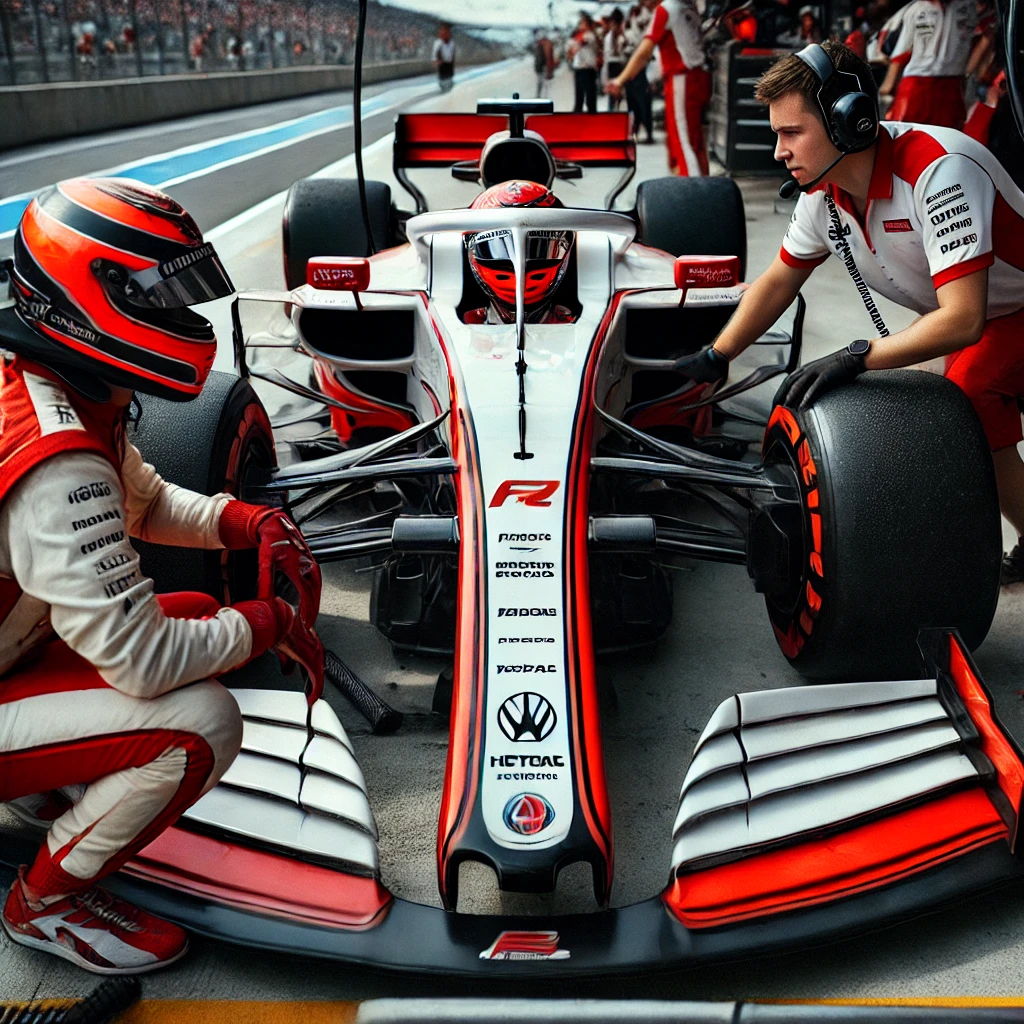
AI/ML or not?
AI gets a lot of press these days. This has created a desire to label products AI, even when this is not justified. Unfortunately, it's sometimes difficult for unsuspecting buyers to tell the difference and it's easy to to get fooled. It's the Mechanical Turk all over again.
One could argue, that if the system delivers the results, it should not matter what the algorithm is. This is correct as far as it goes, but it misses the point. The reason we use AI/ML is that it delivers where previous solutions failed. That's the only reason to use AI. Thus, selling a traditional automation system as AI is like selling fool's gold.
In NDE, automated defect recognition (ADR) has a long and prosperous history. Starting from simple amplitude thresholds and later developed to more elaborate rule-bases systems, these have worked well for simple inspections, e.g., in manufacturing industry for decases. The problem is, that they fail with more challenging inspections. They fail with varying geometry or material conditions. They fail with variability inherent in most important inspections. These required human evaluation, until deep learning and AI finally enabled automation of even the most difficult inspections. AI provides robust automation and can handle the variability where traditional ADR fails.
Now, because of the AI hype, there has been companies that have a well-functioning rule-based system on a simple inspection and they have started to market it with AI. There might be a little bit of real AI added on top of a rule-based system and so it might be somewhat justified. Either way, I think this is rather benign. After all, they have a working system and they are marketing it to a specific inspection task where the traditional systems work and you don't need AI.
However, it's getting worse. I was recently in an AI group meeting, and a prominent NDE vendor miss-presented their rule-based traditional ADR systems as AI. This was in context where the rule based systems are known to fail. These people should now better.
So, I guess the lesson here is you'll need to try before you commit. The true AI systems will typically require tuning/re-training to your data, so you'll need to have some data for the vendor to tune their models and additional hold-off data that you'll use to evaluate the provided solution. If you have specially tricky cases, these should be in the tuning set for best performance. The vendor should also do their own performance evaluation and model validation, so you should also ask them to provide documentation on model performance assessment.
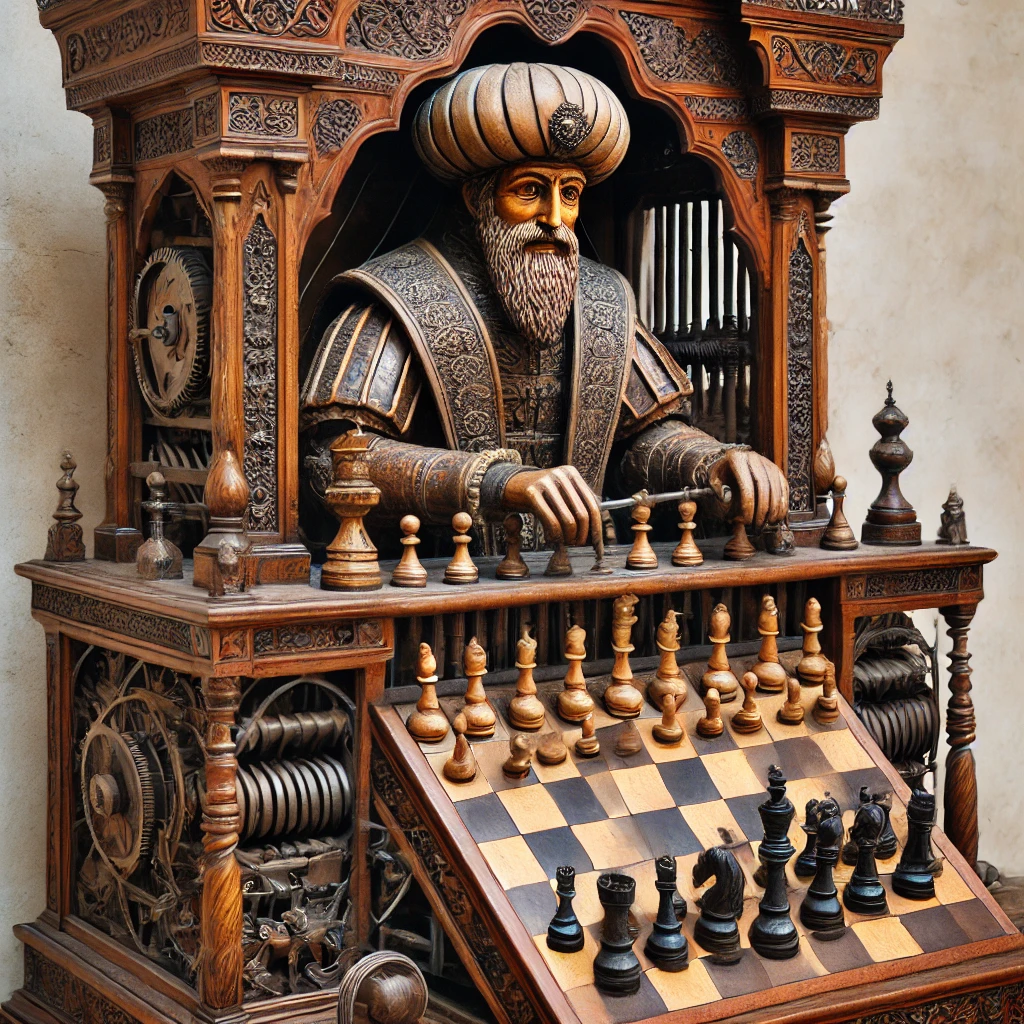
Hard problems first
At Trueflaw, we tend to tackle the hard problems first. We started AI for NDE in the Nuclear and aerospace industries, not because they were easy, but because they were hard. AI being a new technology, building trust was a key to success. Showing success in these highly regulated and safety-critical industries is a good way to show AI can be trusted.
This strategy has worked very well for us. The references are there now and we are seeing NDE at the forefront of qualifying the use of AI in safety significant applications. The flipside is, however, that sometimes we don't talk enough about the easy stuff. There's a ton of industrial applications that can benefit massively from using AI to make inspections more efficient and reliable. Now with the hard problems solved, these are straightforward and easy for us to deliver. Think automated weld lines in pipe factories (UT/RT), boiler weld visual inspection, wind turbine tower weld UT, automotive inspections, robotized tank inspections, etc. These are just a few examples of inspections where it makes no sense any more to continue evaluating data manually.
So, here's a quick summary of how easy it is to integrate AI to your current inspection system and what's needed from you.
The solution
The input to AI is raw NDE data, direct stream of files (.dcm, .nde, .UVData, .opd, .rdt, etc.). You provide the input by copying the file to a shared drive location (either manually or automatically) or streaming data directly for real time applications. The processing can be done locally with the TrueflawBox edge computing hardware for speed and securigy or it can be done in the cloud to share data between multiple sites.
The output is:
- automatically annotated data,
- interactive report with indication list and representative measurements and data plots for final evaluation and/or
- streamlined application for data evaluation.
The end result is, that you save 90% of data evaluation time and your inspectors can focus on the parts of data that actually matter.
The tailoring
For best performance, the solution is almost always tuned for the customers. While there's great similarity between the different customer solutions, we want to make sure that the models are validated for the specific conditions and can deliver the expected performance. This is not a big development project, and is certainly worthwhile. For this we need time and data. You'll need to provide data for 20 flaw indications of each flaw type of interest, and roughly 10x unflawed data. This amount of data is typically something customers already have from test blocks or field data. If this is challenging or you're missing a crucial rare flaw type, we can also manufacture artificial cracks to complement the data. Currently, it takes us 3-6 months from data to delivery.
Sometimes we do see new applications that require more significant development. This is also OK. It just takes a bit more time, up to 12 months.
Cost
On completion, we licence the solution to you on a yearly basis. The cost varies depending on the solution; a good rough estimate would be 35k€/a.
For small tailoring/tuning (~80% of the cases) there's no upfront development cost, just the yearly license. However, you do need to commit to licensing the model for at least one year, provided that the solution meets the agreed performance criteria. For more involved projects (~20% of cases), typically involving customized integration to existing systems or very specific NDE data, there is an additional development cost.
We've worked hard to make AI for NDE reliable, easy and accessible. I think we've succeeded quite well. For any inspection with substantial volume, this should be an easy decision to make. To get started, message me or one of our experts: Sonja, Oskar, Topias, Oskari or Goncalo.
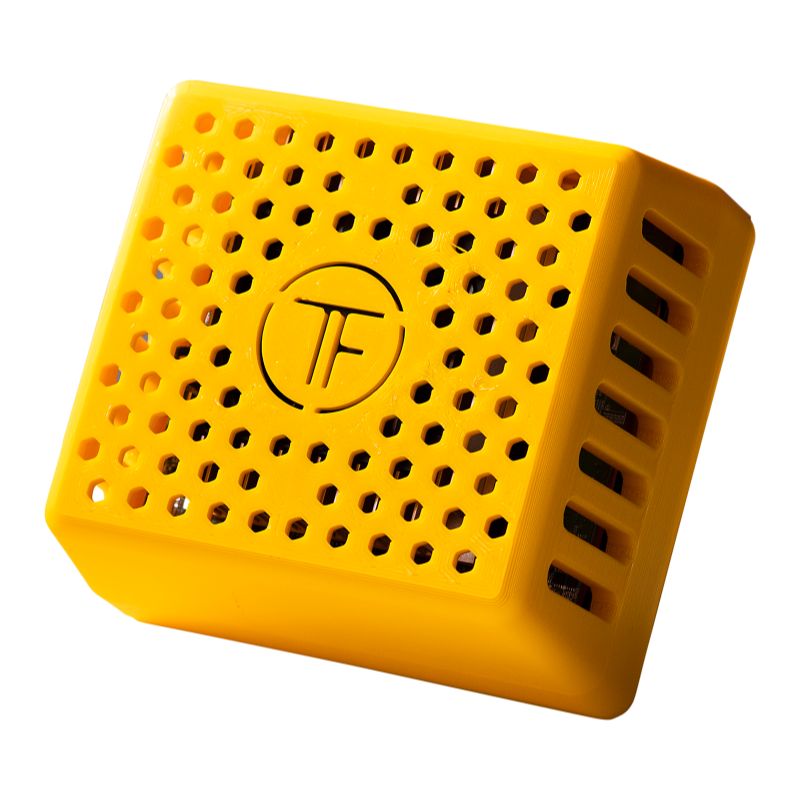
If it can be inspected, it can be automated.
That's where AI for NDE stands today.
Next week, the Nordic Welding Expo kicks off in Tampere. To showcase the latest AI for NDE advancements, we partnered with the welding experts at Suisto Engineering Oy. They welded a tube for us. We made some cracks. We acquired PWI/TFM ultrasonic data with the wonderful Peak NDT Ltd. LTPA. We trained a deep learning network to find the flaws for us. Small flaws in noisy austenitic material, too small to detect with a simple amplitude threshold. Finally, we put the model to the Trueflawbox and connected it to the LTPA for some real-time AI magic.
For those, who cannot make it to Tampere, here's a video showing the working demonstration. Ultrasonic scanning with real-time PWI/TFM reconstruction and AI defect detection.
If you're at the expo, be sure to make your way to booth A80 and talk to Oskar Siljama or Sonja Grönroos and see how to take this opportunity and turn it into a concrete tailored solution for your inspection.