AI for NDE
FlawML is a revolutionary automatic NDT data analysis solution based on machine learning. It combines human-level inspection performance with the speed and repeatability of automation. FlawML offers defect recognition, data quality assessment and customized reporting, seamlessly integrated to your workflow.
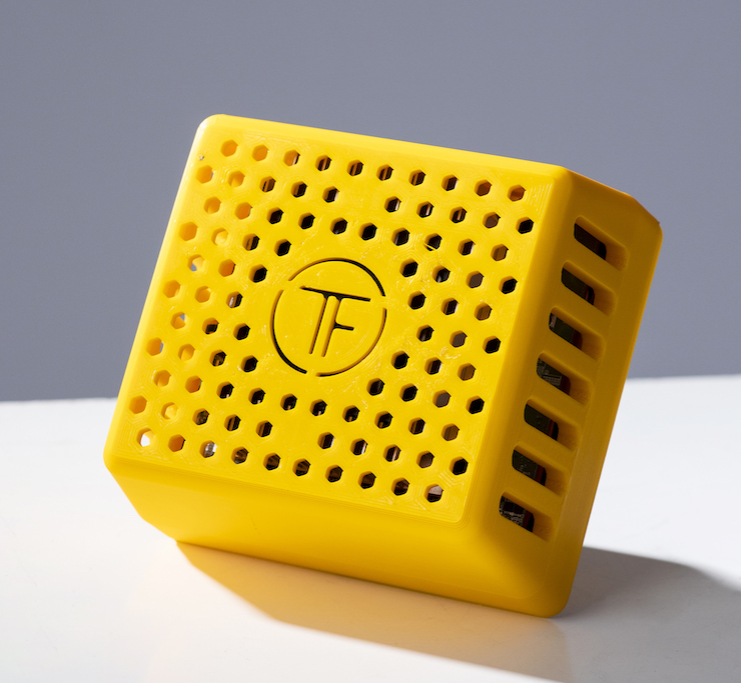
Radiography
FlawML is a highly capable AI assistant for radiography. Automatically detect, measure and classify weld defects. Save time evaluating image quality with automatic recognition of image quality indicators (IQIs) and signal-to-noise ratio (SNR). Organize your reporting with lead letter recognition. Compatible with DR and CR.
We are working with Meyer Turku and DEKRA Finland to bring ADR to shipyard weld inspections! How does it work and how did we get here?
In the Meyer Turku shipyard, a large volume of DR-images are captured daily to ensure weld quality. We were sent a dataset containing these images, which were used to train an AI-based algorithm.
The software is packaged in TrueflawBox, our edge AI solution, giving the necessary computational power in a secure local installation. It automatically processes images as soon as they are acquired. The results are marked on the images - no changes to the inspection procedure are needed.
Features:
- Detect and mark indications according to customer needs
- Measure signal-to-noise ratio (SNR)
- Check image quality indicators (IQIs)
Key benefits:
- Helps the inspector focus on indicated areas
- Makes image quality assessment faster
Next up:
- Classifying defects in standard categories
- Automatic real-time monitoring during manufacturing
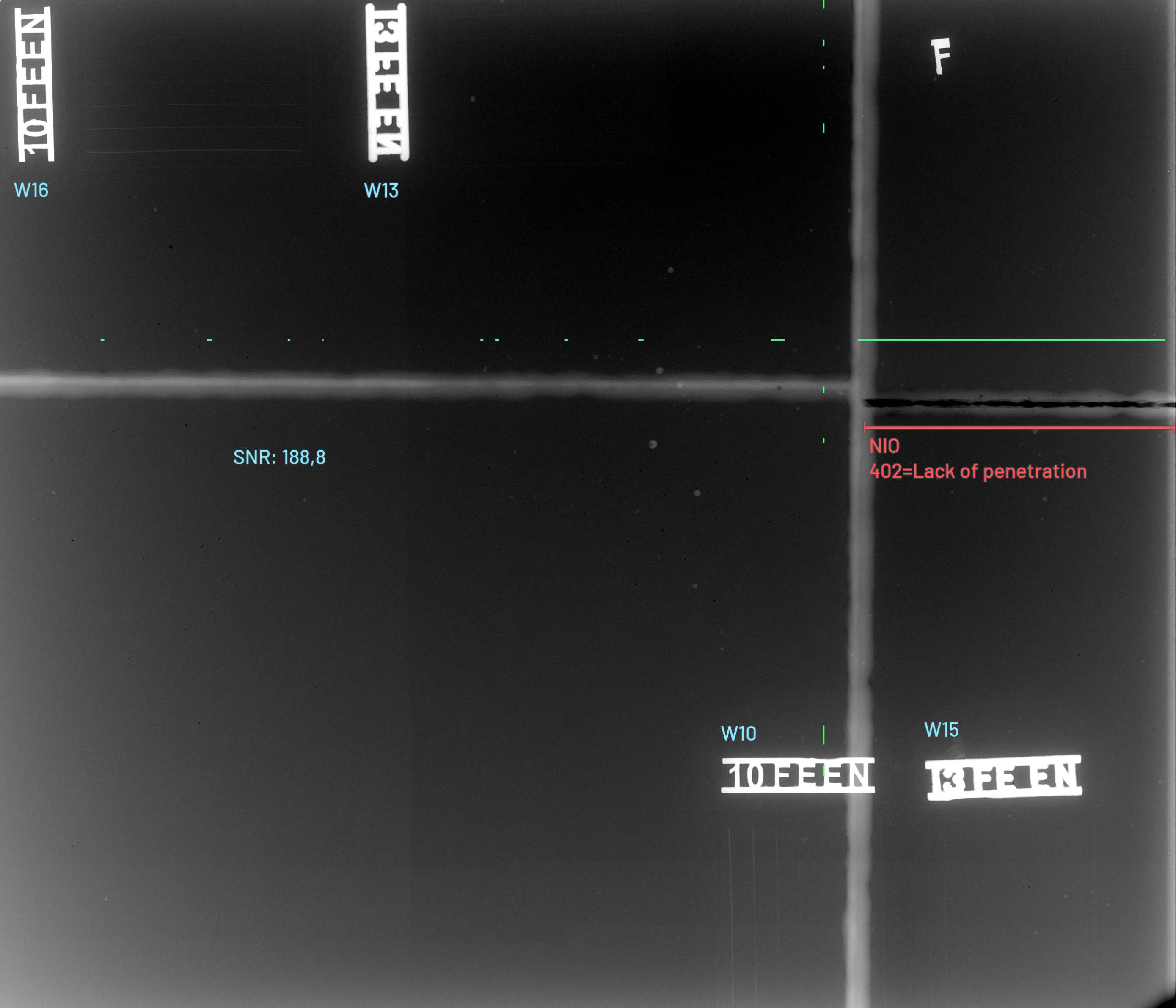
AI can read IQIs and measure SNR in radiographs
X-ray images can go wrong if the imaging parameters are incorrect or there is a fault in the equipment. This is why inspectors use image quality indicators (IQIs) and measure the signal-to-noise ratio (SNR) to check that the data is fit for inspection. Checking for quality, however, takes additional time for every inspected image.
We’ve taught an AI to detect IQIs anywhere in a radiograph, after which the visibility of the IQI wires can be calculated automatically. For weld inspections, our AI detects the weld to automatically find a suitable spot for calculating SNR.
The result? You will know if your image quality is good before even looking at it!
We foresee a future where the inspector’s valuable time is not spent on rudimentary tasks, but rather on the inspection itself. AI is the key enabler for this. Thank you to Meyer Turku for providing the image – more on our collaboration later!
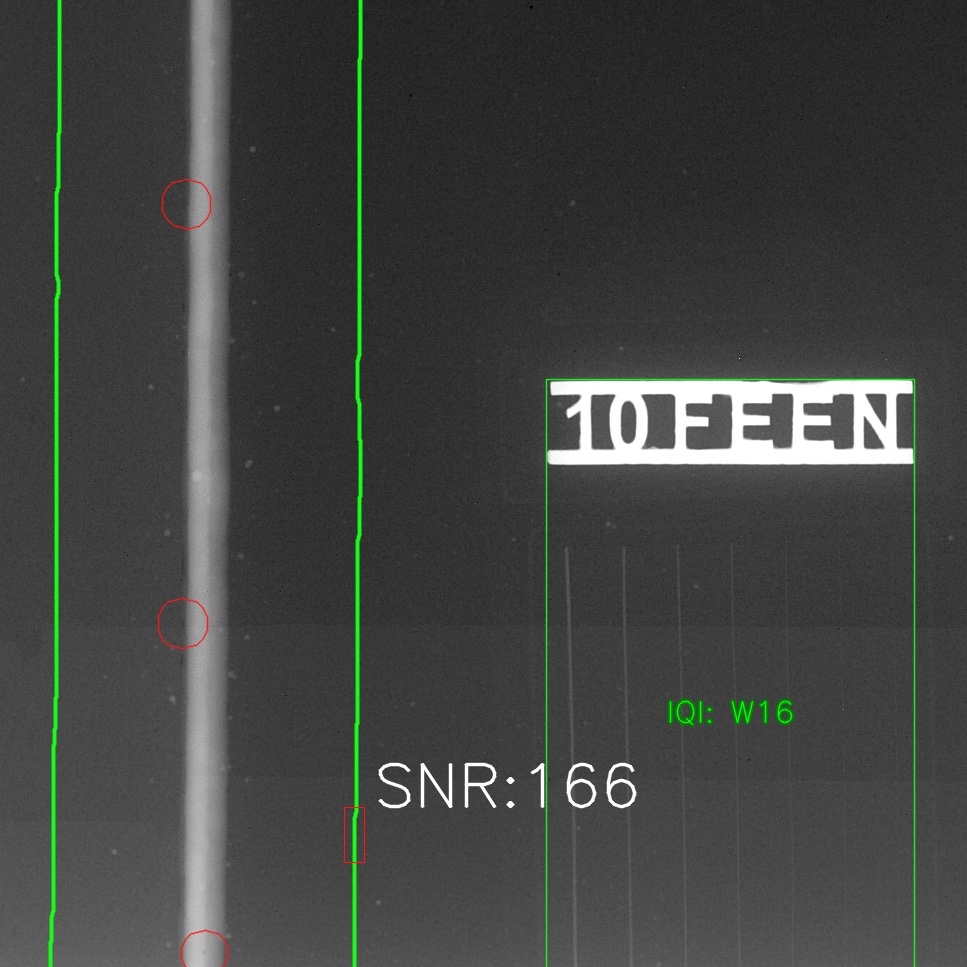
Ultrasonic testing
Mechanized UT generates massive amounts of data, often difficult to interpret. Streamline inspections by prescreening with AI. Get a second opinion on highly critical evaluations. FlawML works with different forms of UT: Pulse echo, phased array or time-of-flight diffraction.
Another AI field trial together with EPRI is coming to an end at a US nuclear power plant.
This field trial was for supporting AI for the difficult dissimilar metal weld (DMW) ultrasonic inspections. Due to the complex and noisy material, the inspection uses phased array ultrasonics with high number of channels to provide the needed inspection reliability. This also creates a lot of data for the inspectors to go trough. AI can evaluate the data and quickly highlight regions of interest to make inspectors job faster and more reliable. It also allows the utilities to quickly get preliminary results that allow them to move forward quickly.
The process of using AI is very straightforward: you take the scanned data file and copy it over to Trueflawbox, which automatically begins AI processing. This is the same data file the inspectors use directly, no conversion needed. The box processess the file in some seconds or minutes (some of these files are over 5GB!!) and out comes an interactive report. The attached video shows an example from test sample data (no plant data). The inspector can then look through the indications directly in the report with readymade evaluation views and, if necessary, go to the raw data for further confirmation. The AI calls are also made available in the native software, so going deeper into the data is easy.
Field trials like these are crucial for a number of reasons. They allow us to test models with real field data, something that is not usually available. They provide opportunity for site personnel and other interested parties to observe and get acquinted with the process. This time, we had people from site, inspection vendor, regulator, and a couple of national labs pop in and look at what we are doing.
We have successfully installed our FlawML machine learning unit to Valmet Karlstad facility, ready to help the inspector in analysing ultrasonic data. Setting up the unit was easy, just plug it into the target computer and the unit is ready to go.
We are happy to be able to support Valmet at being at the forefront of utilising machine learning in NDT. Thank you for the enjoyable collaboration!
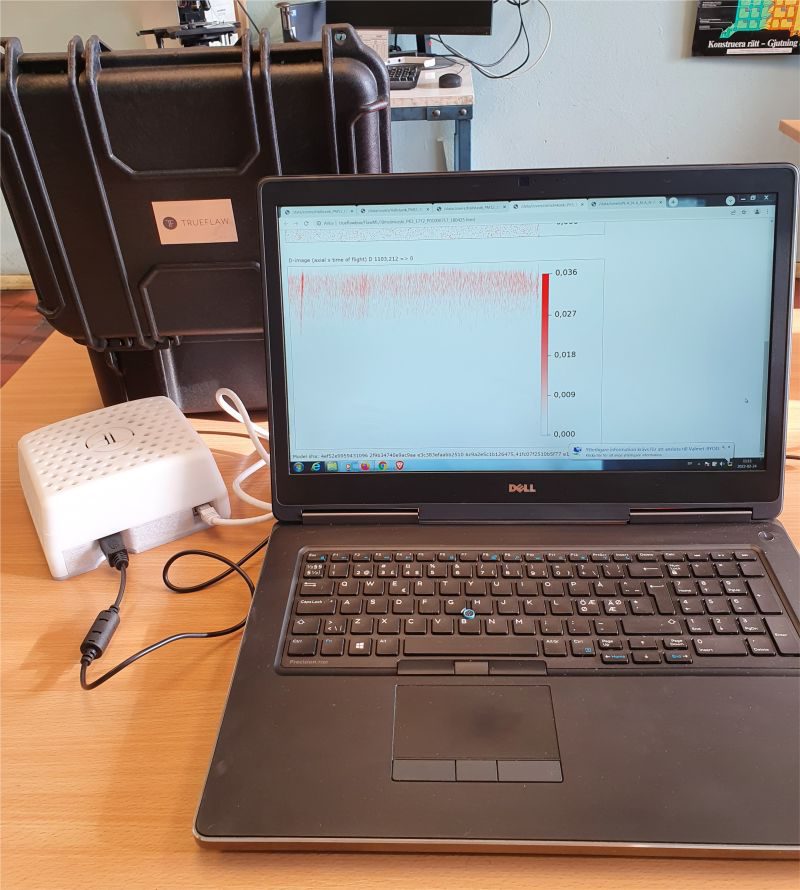
Visual testing
Enhance your image-based or real-time video inspection using FlawML.
Visual inspection is an effective and important inspection method. As with many inspection methods, it takes continuous attention and can be tiring. Machine learning can help highlight potential flaws and ensure they get the inspector's attention.
This model was trained for inspection of nuclear reactor pressure vessel internals. It was field-tested in Olkiluoto power plant in spring 2022. It provides real-time evaluation of video feed for these inspections.
The model generalizes well and can find cracks in other video material quite well. This was demonstrated at the EPRI NDE tech week a couple of weeks ago: we wired the model to video feed from a USB-microscope and provided an aerospace weld sample with Trueflaw manufactured flaws as a test samples. The visitors at our booth could move the sample under the microscope and see the cracks highlighted in real-time as they came to view.
This video was shot using the same set-up. It shows Inconel superalloy weld sample moved under microscope and real-time highlighting of cracks using machine learning.
TrueflawBox
Powerful, secure, and easy to deploy. For the most secure applications, choose TrueflawBox, our standalone Edge AI unit. 32 TOPS of AI performance for fast analysis. Connect to your local network or directly to a PC – no internet connection needed. Customized installations to fit your workflow.
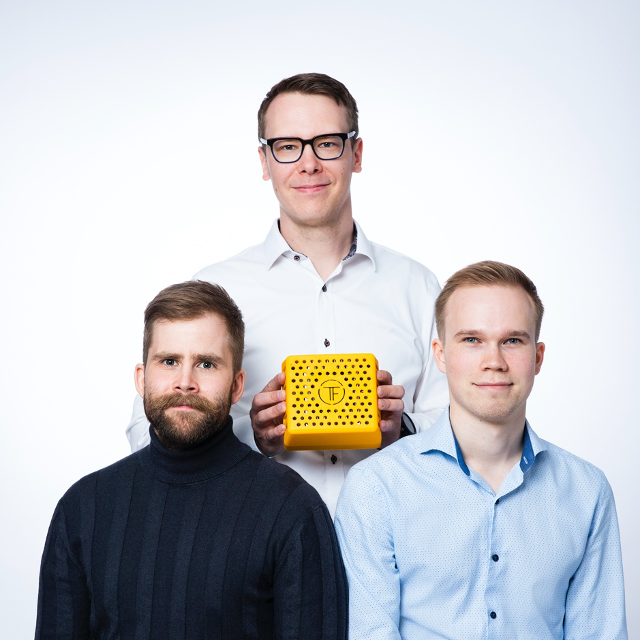
FlawML Web App
For ease of use anywhere at any scale, choose FlawML Web app. Connect to your company data via Microsoft Sharepoint, Jira, Power BI or other platform. Inspect, report and archive in one step - We offer fully featured inspection software on the Web. Use our secure servers or a cloud provider of your choice.
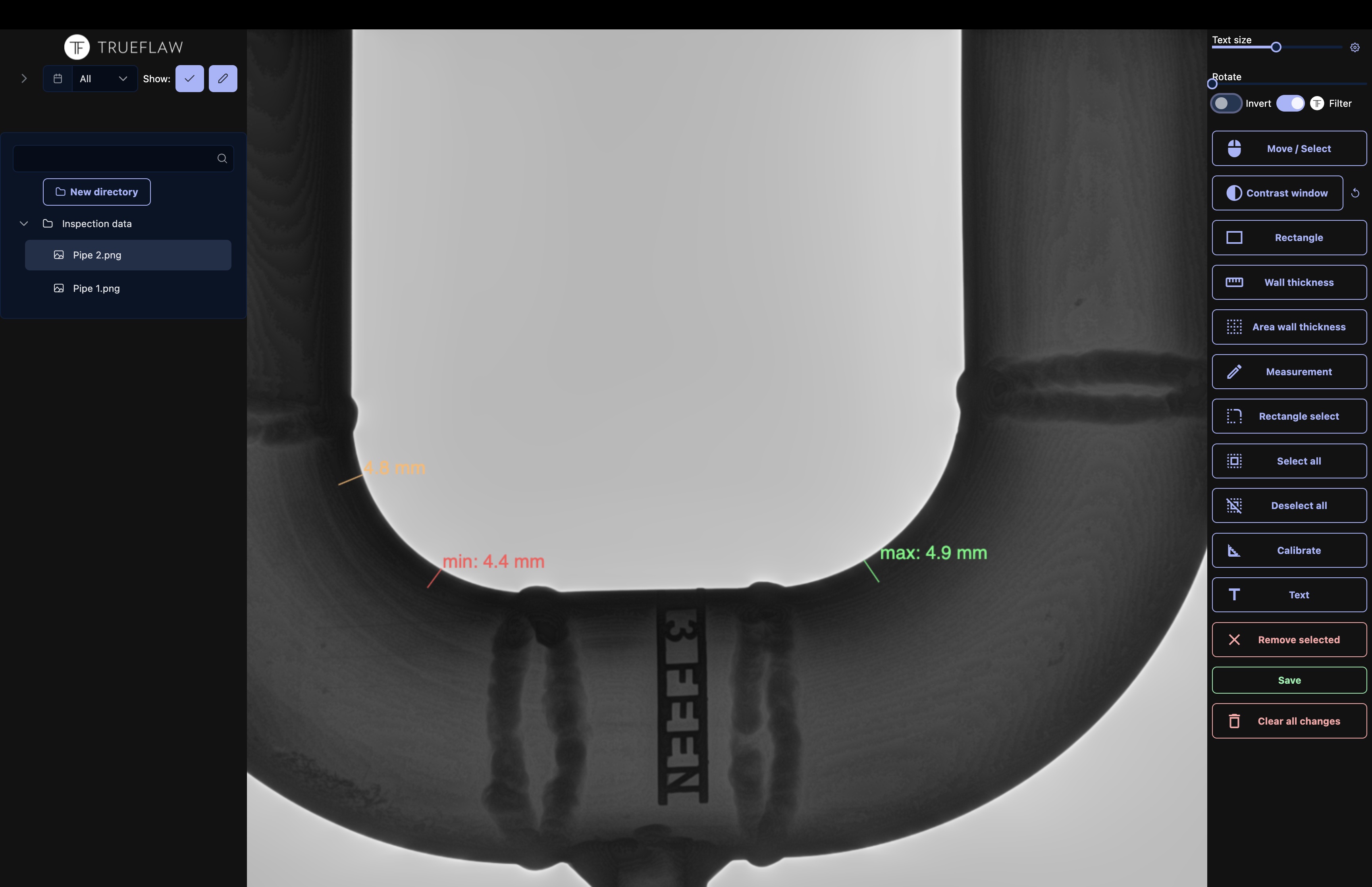
The technology behind FlawML
Trueflaw’s eFlaw virtual flaws allow us to train AI algorithms with less data. For crack detection, we can manufacture physical flaws for additional data. Our expertise in probability of detection services gives an edge at validating AI models for inspection use.
Boosting AI performance with flaw manufacturing!
There is often scarce number of flawed samples available in NDT data. A lot of flawed and unflawed samples are required to train AI model with human-level performance. The data sets should also have a lot of variability to cover the entire use case. There are no shortcuts in data for training a high-performance AI model.
We at Trueflaw manufacture real cracks using thermal fatigue. These cracks can be used for training, reliability assessment and to confirm that NDT procedures meet their requirements. These samples can also be utilized to train AI. Real cracks are unique and possess natural features that are difficult to copy or simulate, making them the best source of data. Next up are a few case examples.
-
Nuclear PAUT
We have trained AI model for phased array ultrasonic testing (PAUT) application to detect flaws from dissimilar metal weld by manufacturing more flawed samples to a data set with limited number of flawed samples. This introduced additional real variability to the data set, boosting the performance of the AI model. We achieved much better results on the target test data. -
Aerospace radiography
The same principle was used successfully for radiography of aerospace components, explained in “Automated defect detection in digital radiography of aerospace welds using deep learning”. You can find it here. We manufactured cracks onto plates of the same material and similar thickness as the actual components. They were then radiographed and transferred virtually. Trained with only virtual flaws, the AI found natural cracks in the real components. -
Train axle UT
Thermal fatigue cracks were also an important part of developing out first AI system for the Helsinki metro train axle inspection. We manufactured cracks directly onto a real train axle to get the most representative data.
Virtual flaws help train ADR for radiography
What is a virtual flaw? Simply put, it’s a defect taken out of background. With virtual flaws, we can create endless flawed data, often a rare commodity in NDT. Virtual flaws help train our FlawML machine learning ADR solutions, that learn by example like people do.
In a research article in collaboration with GKN Aerospace Sweden AB and KTH, now accepted for publication, we studied the effects of using virtual flaws for training a machine learning algorithm to find defects in weld radiography.
We started with a dataset containing sufficient material for training the algorithm, and then made the task more difficult by limiting learning to smaller and smaller subsets of the original dataset. We found out that as datasets become smaller, adding virtual flaws significantly improves the accuracy of finding defects.
To add capabilities in finding cracks, critical to safety but very rare in this inspection case, we manufactured thermal fatigue cracks using Trueflaw technology to sample specimen, took radiographs of those and collected virtual flaws from the radiographs. We were able to detect naturally occurring cracks by only training on virtual flaws.
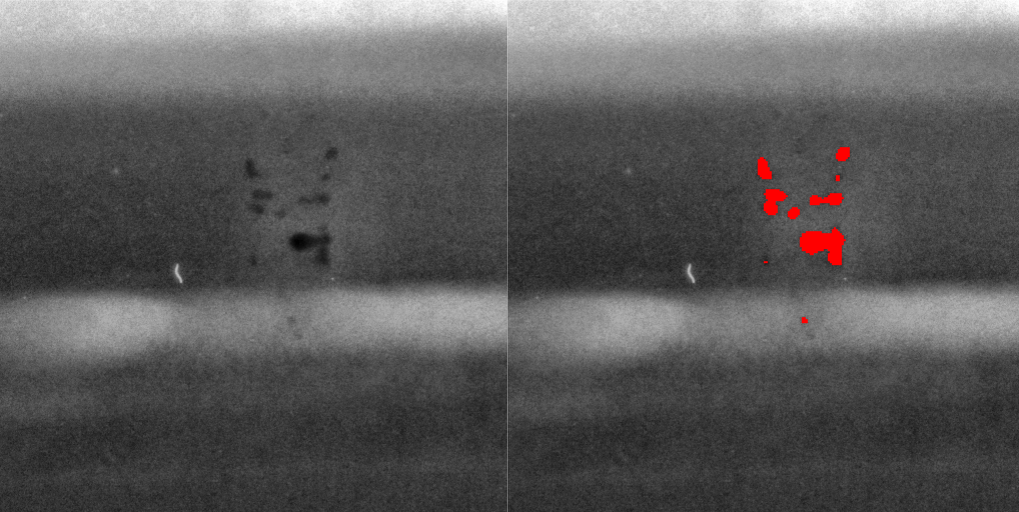
Get started!
Contact us for an assessment on your case.